Measure What Matters Part I: Media Mix Modeling (MMM)
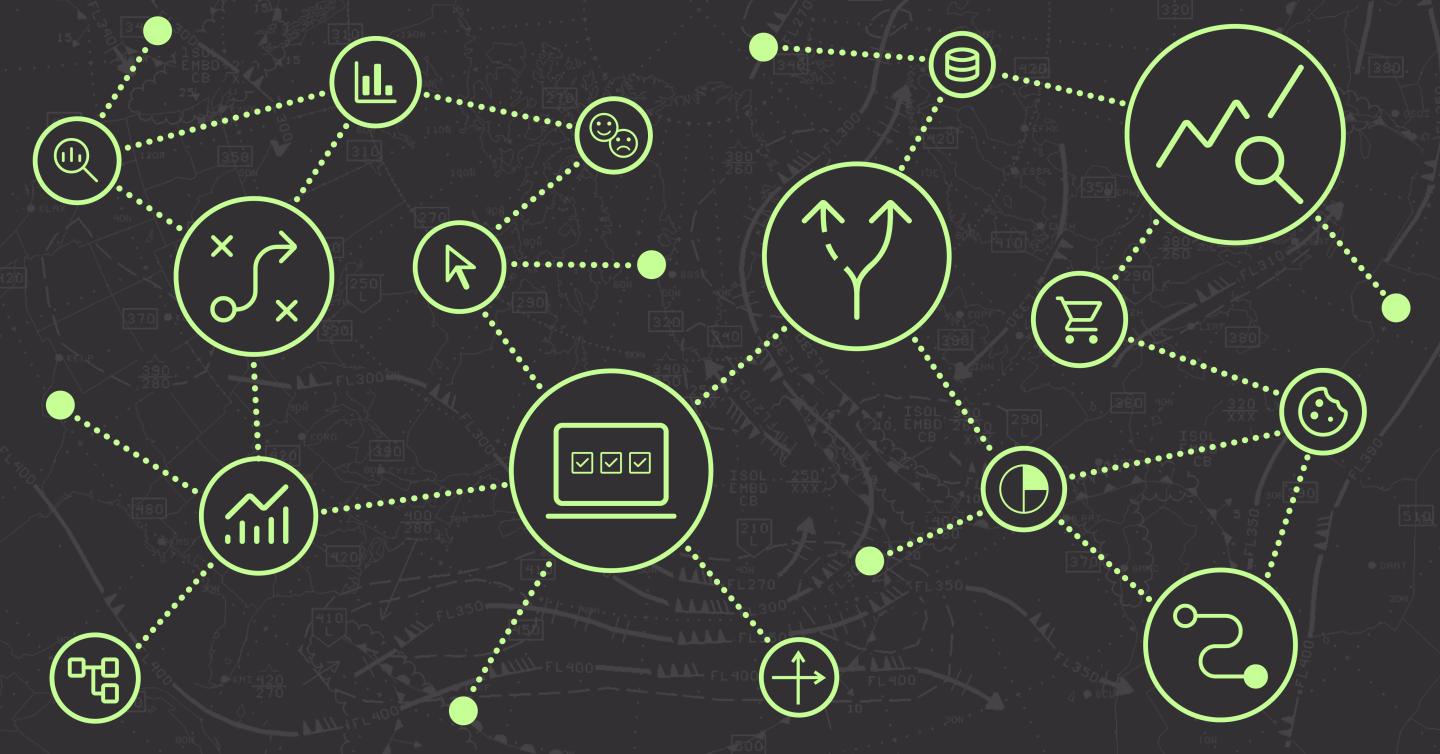
As an advertising agency with chops in paid media, public relations, search marketing, affiliate marketing, social media, influencer marketing, creative and content, and data analytics, we get measurement. In this content series, we’re delving into the nuanced and multifaceted measurement process to help marketers understand which measurement solutions are best for their brand and how they can be integrated into a holistic measurement strategy. Read more about why it’s important to Measure What Matters in our introduction piece.
First up, we’re diving into the world of Media Mix Modeling (MMM), which uses a variety of statistical methods to correlate patterns in channel selection, spend, time and more to sales while involving no direct user tracking.
What is Media Mix Modeling?
MMM is a statistical analysis that takes large amounts of historical data, typically six months to several years, and (ideally) indicates which marketing tactics (online and offline) are contributing most to business success. Further, MMM can identify the optimal level of spend for each tactic and forecast spend scenarios across an entire media mix.
What is the history of Media Mix Modeling?
Media Mix Modeling is one of the oldest forms of media measurement. In the ’60s and ’70s, media mix models were created to measure the “unmeasurable” using regression models (statistical tools that correlate relationships between variables) to show how spend in traditional media channels (TV, radio, print, etc.) contributed to business outcomes. Given the long flights of paid media and large amounts of data required for these statistical methods, early MMMs were not nimble and often took months and years to produce findings.
With the arrival of digital advertising in the late ’90s and early 2000s, marketers incorporated even more channels into their MMMs. However, the fast-paced and trackable nature of digital advertising posed challenges to relatively plodding MMMs, which still took months to yield results. So, marketers pivoted to using tracking-based measurement (e.g., multi-touch attribution or MTAs) that produced direct insights into how advertising was contributing to the success of their business.
However, over the past five years, cookie blocking, privacy regulations, walled gardens, and a propensity by users to not click ads have made tracking clicks harder than ever. This has led to a renaissance of MMMs which, by nature, do not require any user tracking to function. Additionally, MMMs have been boosted by technological advancements in machine learning and the availability of even more data for input. Legacy players in the space are now joined by nimble, AI-powered models and open-source solutions from media giants like Meta (Robyn) and Google (Meridian).
What questions does Media Mix Modeling answer?
Media mix models primarily answer the question: in which channels should media budget be invested and how much should be spent?
MMMs correlate historical media spend and other factors to business outcomes, indicating which actions were most associated with sales and revenue, among other metrics. While users can look at past results to inform decision making, most MMMs also include scenario-planning tools that allow marketers to forecast results based on hypothetical budget allocations across the entire media mix.
Additionally, forecasted results are not based on aggregate cost performance but, rather, the marginal return of spend, answering the question: how much money should be invested in each channel? This considers the fact that, as spend in a tactic scales, it’s unlikely to maintain consistent cost efficiency. In other words, incremental dollars will produce fewer conversions at higher budget levels.
Advanced models can inform other questions like: which marketing tactics produce results when launched simultaneously? Or how long does the influence of paid media last?
What does Media Mix Modeling measure and output?
- Modeled Return on Ad Spend (ROAS): revenue earned for each dollar spent on advertising, estimated by model
- Modeled Cost per Acquisition (CPA): the direct media cost of driving a conversion, estimated by model
- Modeled Customer Acquisition Cost (CAC): the media cost of acquiring a new customer, estimated by model
- Cost curves and budget recommendation: a chart showing predicted ROAS or CPA based on media spend by tactic, used to identify the point of diminishing returns on media spend to identify optimal investment.
- Decay: a representation of how the effect of media tactics diminishes over time
- Interaction effects: a representation of how combinations of simultaneous media execution drive outcomes
- Seasonal patterns: correlations between time of year and outcomes
What does Media Mix Modeling not measure or output?
- Immediate measurement on new tactics: since MMMs use historical data, they take time to return results on new tactics as results are collected
- Path to conversion: the ordered touchpoints of a customer’s purchase journey
- Fractional attribution: partial, deduplicated conversion credits to media tactics
- Incrementality: the percentage of platform-attributed revenue causally impacted by the media tactic
- Brand health: qualitative measurement on consumer sentiment towards a brand (e.g., awareness, consideration, favorability, etc.)
What is necessary to implement Media Mix Modeling?
- Organization
- Leadership buy-in, implementation plan and decision-making protocol
- Domain knowledge of media mix and business
- Ability to incorporate longer lead time to insights (up to several months)
- Personnel
- A trusted modeling partner or in-house personnel with statistical and software expertise
- An internal project and product owner(s)
- Media agency with MMM experience
- Data
- At least several media channels and tactics
- At least one but, ideally, several years of historical media performance and sales data
- Accurate, complete and clean
- Well-organized and documented
- Privacy-compliant
- Technical Resources
- A trusted modeling partner or in-house software resources and data infrastructure
How can Media Mix Modeling results configure and validate other measurement tools?
- Post-purchase surveys
- User bias validation: Results from an MMM can validate customer responses in post-purchase surveys. By comparing MMM results to responses from customers about where they saw advertisements for the brand, we can ensure the validity of both sources of information. Additionally, this check increases the validity of other survey responses, with the assurance that they are not over indexed on users from certain channels.
- Multi-touch Attribution (MTA)
- Create constraints: an MMM can identify tactics that are not receiving the correct amount of credit within an MTA. This may reveal tracking issues or problems with the model configuration.
- Decay: time decay on the effectiveness of a media tactic can be incorporated into and MTA result by discounting the value of a touchpoint depending on the time between it and the conversion.
- Channel budget and role: an MTA can identify which channels perform best at various stages of the customer journey (high, mid or low funnel). Combining this with the predicted, optimal budget of the channel from an MMM can help marketers assess if the investment in that channel is feasible
- Incrementality Testing
- Causal effect: Incrementality testing is one of the only ways to measure the causal effect of media tactics on conversions. That is, the number of conversions that wouldn’t convert if the tactic were paused. These results can ensure that an MMM isn’t incorrectly correlating media investment in one tactic to sales.
Backbone Media Mix Modeling case study
Challenge
One of Backbone’s clients, a fast-growing home-goods brand, needed a better understanding of how their channel investments contributed to growth across all sales channels. Relying on tracking pixels and last-click attribution from their digital channels didn’t identify which channels were driving growth and the optimal amount of spend for each.
Solution
By implementing an MMM, Backbone and the client were able to see the effect of their media investment on both website and online retailer sales. This informed strategic adjustments, offering a complete view of return on ad spend (ROAS) and the ability to scenario plan for an optimal media mix.
Results
Results indicated that the true ROAS on the client’s Meta spend was 3.5 times higher than the platform reported. Using this information, they increased spend by 111% during a sale period, which contributed to an 86% revenue increase and surpassed internal revenue forecasts by 41%.
Overall, Media Mix Modeling is an essential tool to incorporate in a holistic measurement strategy. Stay tuned for part II of this series: Incrementality.
Please reach out to info@backbone.media with questions.